Share
As one of the Centre’s 2019 Data Fellows, I was tasked with researching the elements for a peer review framework for predictive models in humanitarian response. The desire for quality assurance had been discussed amongst the Centre’s partners at a workshop in April 2019, with the Centre identified as having a unique role to facilitate a peer review process. Over two months in June and July, I explored best practice in academia, interviewed experts and stakeholders, and developed a draft framework for consultation.
Humanitarian decision-makers have called for the increased use of predictive analytics to inform anticipatory action. However, translating the outputs of predictive models for timely and appropriate responses remains a challenge for several reasons:
- First, there is no common standard or mechanism for assessing the technical rigor of predictive models in the sector.
- Second, the development of predictive models is often led by technical specialists who may not consider important ethical concerns, such as the consequences of a false positive (a model output that predicts a crisis when one does not manifest) or a false negative (a model output that fails to predict a crisis that occurs).
- Third, model outputs may not be actionable or relevant for humanitarian decision-making due to mandate, policy, resource, or other constraints.
My research
I was asked to address the following problem statement: how might the Centre facilitate and communicate rigor around predictive analytics for humanitarian action? I organized my research around the following questions:
- What value could be added from peer review?
- What are the necessary components of peer review for predictive models?
- What roles are required in a peer review process?
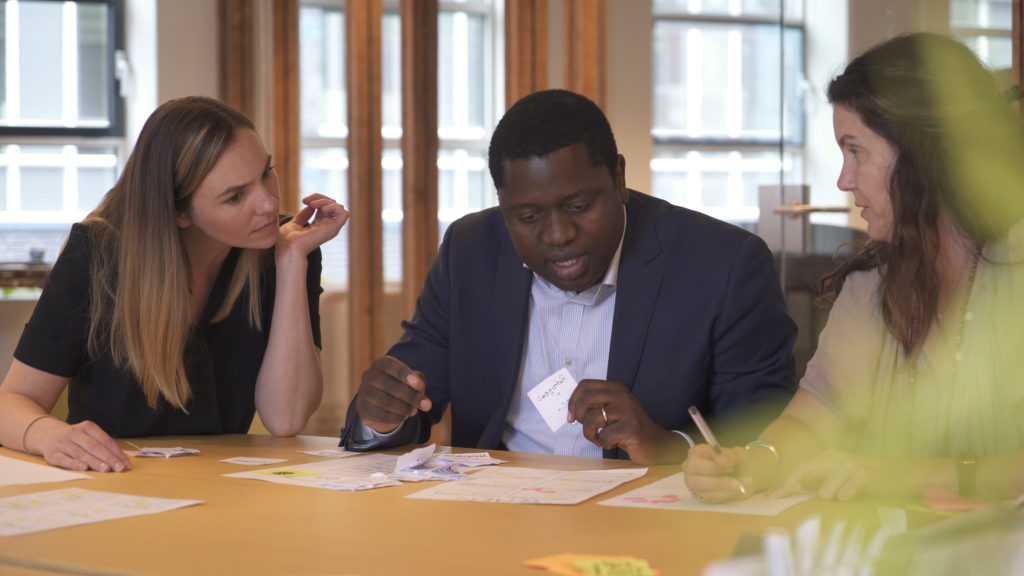
My approach began with a review of humanitarian applications of predictive analytics—what models are being developed or in use across the sector? As the Centre detailed in its April workshop report, the use of predictive models in humanitarian operations is only just beginning. While there are promising models from the IFRC and the World Bank, the work is fragmented across organizations, often poorly funded and can be disconnected from implementation and decision makers.
I went on to research existing peer review processes. My assessment focused on how reviews are typically undertaken and what the process is for getting the results published. In academia, I identified checklists for reporting predictive analytics studies such as the Guidelines for Developing and Reporting Machine Learning Predictive Models in Biomedical Research*. From the private sector, I learned about reporting requirements for insurance companies that propose rates based on predictive models. I also considered steps that have been taken to improve transparency, such as the publication of the peer review history.
I conducted interviews with over 20 experts including data scientists, researchers, ethicists, and decision makers spanning the humanitarian, academic, and private sectors. In these interviews, I learned about the perception of predictive analytics and the culture around model development and use. I heard about the need for careful ethical and actionability assessments rather than the more common technical review. One interviewee referred to the often-used aphorism in statistics: ‘All models are wrong, but some are useful.’ This saying points to the need to consider the uncertainty and reliability of model outputs.
I also discovered new peer review initiatives such as the working group on the ethics of humanitarian data science led by IOM. Additionally, the mathematician and author Cathy O’Neil has created an ethical matrix to evaluate the consequences of model output and inaccuracies from stakeholder perspectives, which we have incorporated into our process with her guidance.
An initial framework
Based on these investigations, I created an outline for a peer review framework for predictive analytics in the humanitarian sector, and then worked with the Centre team to fill in the details. The framework consists of three steps: 1) readiness assessment; 2) model review; and 3) recommendations.
In the first step, the Centre would assess the readiness of a model for peer review. They would work with the partner to understand the model objectives, the crisis setting, and the action that the model output will inform.
In the second step, the model would be assessed against three criteria: technical, ethical, and humanitarian relevance. For this, the Centre would invite experts to engage in the review process. Interested reviewers would submit a brief application and if accepted, they would be added to a ‘reviewer pool’ which would be managed by the Centre.
In the third step, the Centre would convene the reviewers to discuss the findings. A recommendation package would be developed that includes findings across each domain and the final assessment of the model. This package would be shared with the partner privately. The partner may decide to revise their submission based on the findings and would have the ultimate say in whether the results are shared publicly.
The framework includes annexes with templates for each step, including: the readiness assessment; the technical checklist; the ethical matrix; the humanitarian relevance checklist; and the recommendation package.
Review the complete peer review framework here.
My recommendations
Beyond operationalizing the framework, my long-term recommendation is for the sector to invest in understanding the impact of predictive analytics in humanitarian response. An important outcome of such analyses would be a better understanding of the return on investment of developing and using predictive models to inform anticipatory action.
Additionally, I would like to see more transparency from organizations in sharing the cleaned data and code for their models. Related to this, an open-access repository for model protocols, peer review results, and impact evaluations of applied models would further advance the sharing of lessons learned in this rapidly growing field. As a step further, the Centre may consider publishing a peer review journal to share these scientific advances with the humanitarian and academic communities. The journal could cover model development, validation studies, and impact analyses.
My fellowship provided me with an opportunity to apply my knowledge of population health science and my experience with peer review in academia to this important challenge. Peer review offers the humanitarian community an avenue for creating and sharing rigor in a way that maximizes resources to mitigate human suffering. I will continue to follow this work closely and look forward to supporting the Centre’s peer review process and the dissemination of findings resulting from the use of predictive analytics for humanitarian action.
Watch Dani Poole present the results of her fellowship on predictive analytics at the Centre’s Data Fellows Showcase event in The Hague in July 2019.
The Centre’s Data Fellows Programme is undertaken in partnership with the Education Above All Foundation. Learn more about the 2019 Data Fellows Programme, see video and photos from final presentations at the Data Fellows Programme Showcase, and read about the work from the Programme Lead and Data Science (Education), Business Strategy, and Statistics (Disability Data) Fellows.
Thanks you Ms. Poole for this excellent synthesis and tangible way forward for Peer Review related to predictive analysis. Your work and commitment to this topic is well recognized and I am excited to see how we can put this into pro-active practice.